AI and data security: a delicate balance of protection and privacy
As artificial intelligence (AI) tools continue to integrate into various business processes, the intersection of AI and data security has become a critical concern for organizations worldwide. From cloud-based copilots to third-party AI tools, the potential for unintentional data exposure has never been higher. This delicate balance of embracing innovation while safeguarding sensitive information demands careful consideration. In this article, Fellowmind’s data security expert, Frederik Stengaard, highlights key risks and best practices to ensure the safe integration of AI in the workplace.
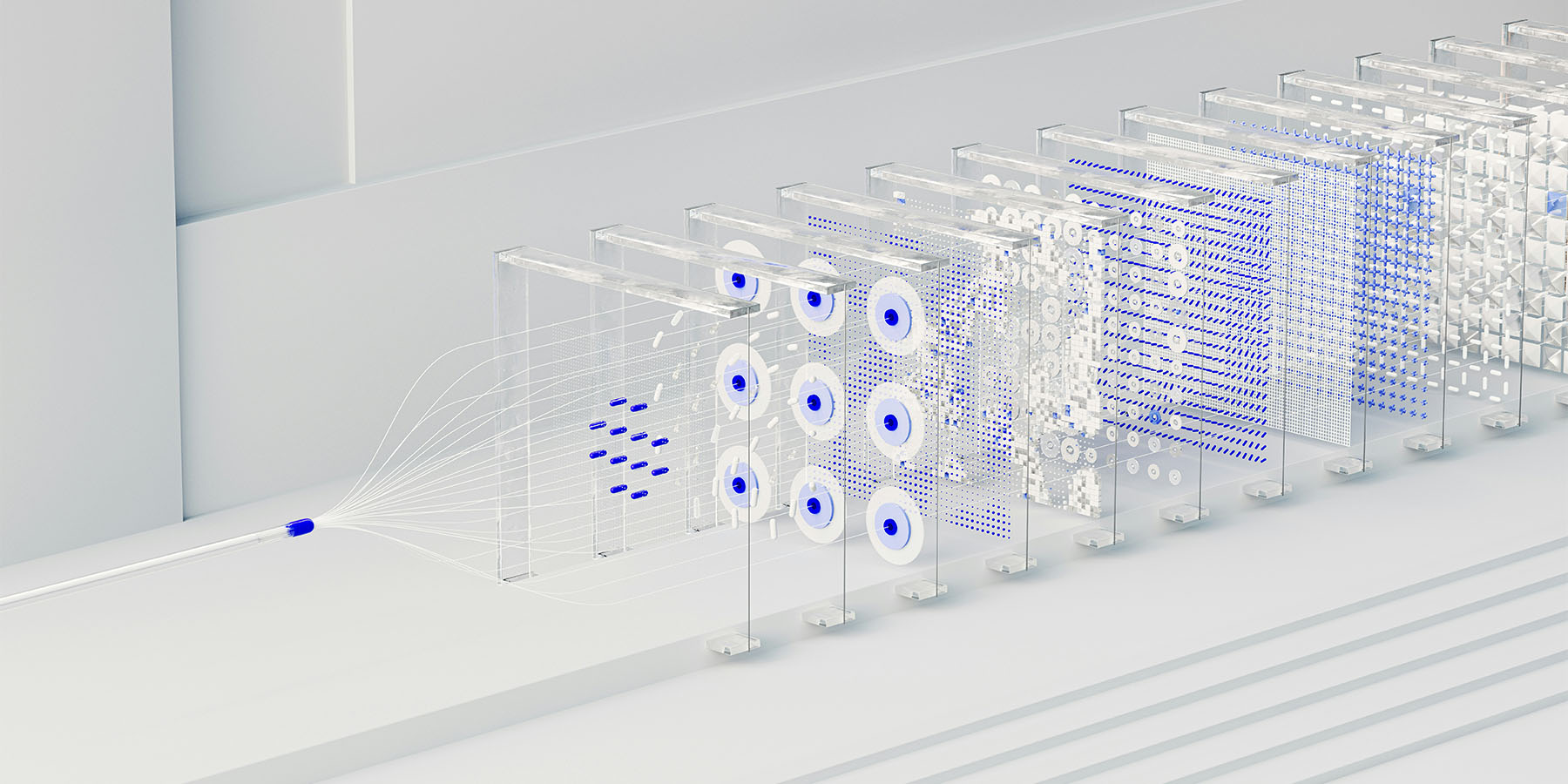
The risks of over-sharing information
Frederik emphasizes one of the most significant risks of implementing AI: the over-sharing of information.
"AI tools, especially copilots, can inadvertently access and expose personal and proprietary data if proper safeguards are not in place," Frederik explains. "This can lead to serious repercussions, from violating GDPR regulations to exposing critical business secrets, such as production methods in manufacturing."
One of the first lines of defence is identifying what data is truly critical to the organization.
"We refer to these as the 'crown jewels' - The data and systems that need the most protection," says Frederik.
For instance, engineering drawings in a file share that feed into a production line are highly sensitive and should be off-limits for AI tools. Recognizing and safeguarding these critical assets is fundamental to a robust data security strategy.
Managing third-party AI tools
Third-party AI tools pose a unique challenge when it comes to data security.
Frederik explains, "Using tools like image-generation AIs without proper data transfer agreements can open the door to potential data leaks."
The lack of transparency in how these tools handle data exposes organizations to risks, particularly when sensitive information may be passed on to external parties without adequate protections.
In many cases, organizations are opting to restrict or prohibit the use of third-party AI services altogether.
Implementing policies that limit what data can be prompted and processed within these tools is essential to mitigating data exposure risks.
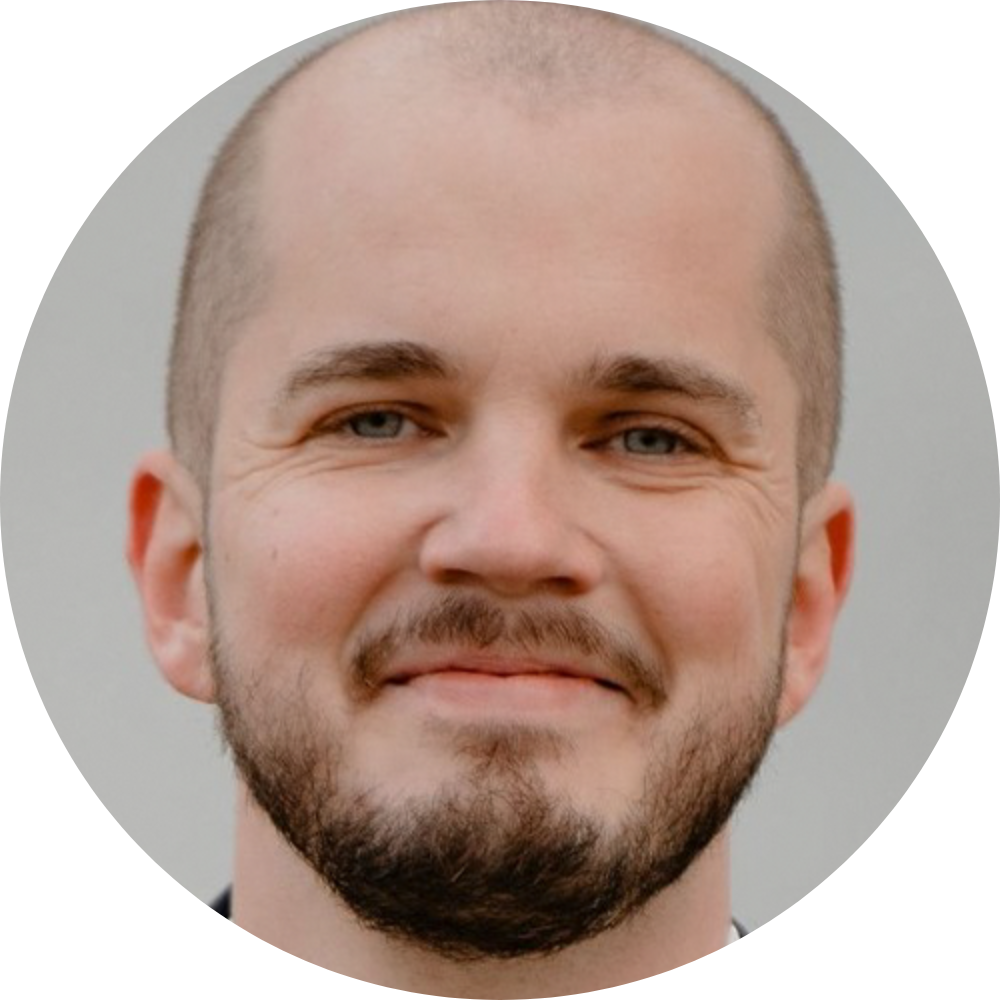
Without such restrictions, an organization's data could unknowingly be processed and stored by external parties, raising serious security concerns.
Best practices for integrating AI securely
When integrating AI into business operations, Frederik outlines several best practices for ensuring data security from a technical perspective:
- Housekeeping: Ensuring that data access controls are up-to-date, and that old sharing links are removed from public environments is essential to preventing inadvertent data exposure.
- Defining data types to protect: By categorizing data into different levels: public, internal, and confidential, organizations can apply specific protections to each class. This classification helps teams understand which data types should be safeguarded at all costs.
- Implementing preventive controls: Tools like Data Loss Prevention (DLP) policies can restrict users from sharing sensitive data with AI tools or prompting AI about critical information. "These tools are essential in preventing users from unintentionally violating data security policies," Frederik notes.
- Leverage the expertise of a trusted partner like Fellowmind: With years of experience in data protection, Fellowmind offers tailored solutions that ensure your sensitive information is well protected throughout the entire AI implementation process. We can help you create clear policies to prevent data leaks and put systems in place to catch any potential security risks, allowing you to innovate confidently while keeping your data safe.
Conclusion: prioritizing data security in the age of AI integration
As AI tools become more common in the workplace, data security must remain a top priority. Protecting sensitive information requires a multi-layered approach. This includes restricting access to critical data and implementing strong preventive measures. By following best practices, such as defining data types, cleaning up existing environments, and managing third-party AI tools, organizations can integrate AI successfully while minimizing the risk of data exposure.
In the words of Frederik, "AI offers incredible potential for innovation, but it must be deployed with caution. Understanding and protecting critical data is the first step in ensuring that AI’s benefits don’t come at the expense of security and privacy."